Hamish Friedlander - RUSH's Head of Engineering and AI
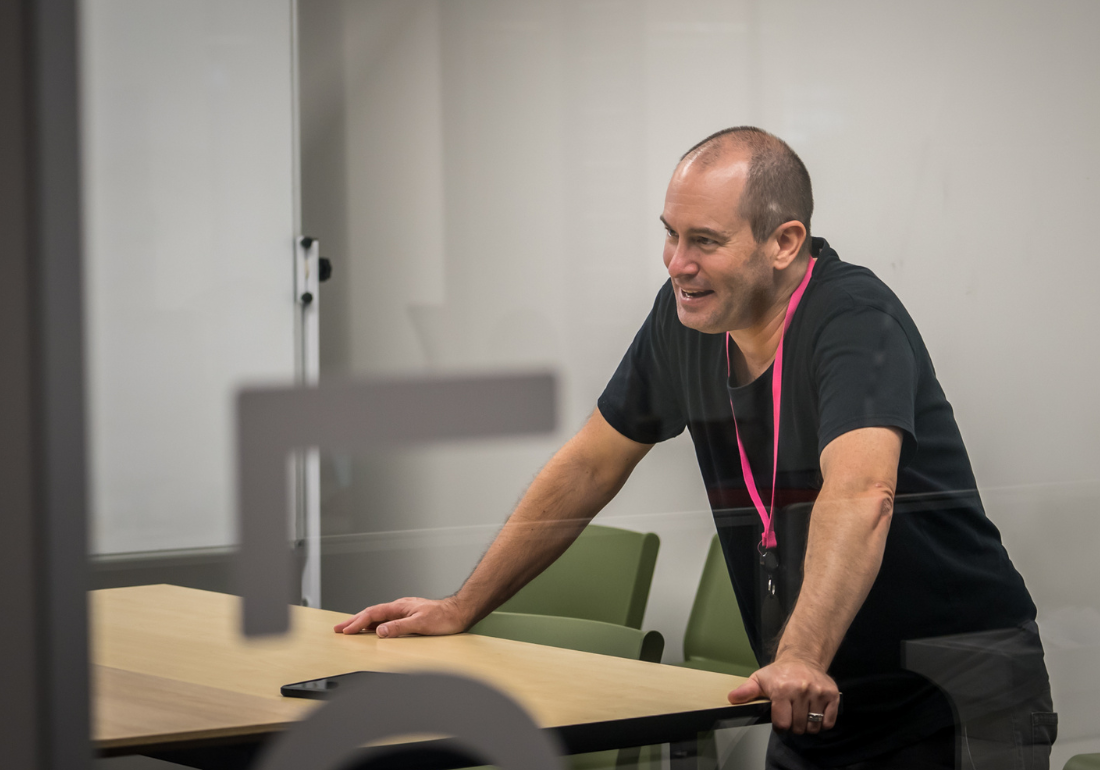
We are thrilled to announce the expansion of RUSH's Hamish Friedlander's role to the new appointment of Head of Engineering and AI.
Hamish, who has been our Head of Engineering for the past three years, expanded his responsibilities in April this year to encompass this crucial AI leadership position. In this expanded role, Hamish will spearhead RUSH's initiatives to integrate cutting-edge artificial intelligence capabilities into its product and service offerings.
In this interview Hamish discusses AI capabilities and the ethical considerations to create impactful solutions for our clients. At RUSH, we believe the future of software development will increasingly involve AI-assisted processes. But fear not - the human-centric core skills of problem-solving and design thinking will remain as crucial as ever.
Alaina: So first off, you are now the Head of Engineering and AI. How does this addition to your role expand your remit for RUSH?
Hamish: I'll speak for myself here. It's still a bit unclear if AI will definitely become a mainstream thing or not. There have been AI winters before, periods where we thought AI could do amazing stuff but then hit roadblocks preventing it from becoming a practical tool for regular use. However, there's about an 80% chance that AI is going to be a major force, just from the billions of dollars that companies like Google and Microsoft are pouring into it.
The amount of money being invested in AI is staggering - Microsoft recently spent $35 billion just on GPUs for AI, which is comparable to what America spent on the Manhattan Project. So a lot of resources are going into AI development.
Alaina: And one of the pretty obvious outcomes of AI, if it does take off, is that it's going to transform what engineering and software development look like, right?
Hamish: That's something I've been saying for a while. None of the code I write now will likely exist in 5-10 years, because just like we went through major transformational shifts in how we interfaced with computers in the 90s and early 2000s - from BBSs to the internet to websites and apps - AI seems poised to be another transformational event that will dramatically change software engineering workflows.
For the last decade or so, mobile has dominated user interfaces. But AI seems like it will shake that up again. So to me, it was recognising this reality that software engineering is going to be a very different discipline in a few years due to AI's impact. We need to start planning and adapting for that inevitability.
Alaina: At this point, because of the investments from big tech companies, AI's mainstream adoption almost feels too big to fail.
Hamish: I think AI is having its best chance yet of real-world success with current technology. But that doesn't necessarily guarantee it will succeed - look at virtual reality. Billions were sunk into VR and while the tech improved over 20 years, it never really took off as a mainstream platform despite the investment, at least not yet.
The current AI models can do amazing things that would have seemed unbelievable just 5 years ago. But there are still constraints around hallucination issues, where the models can't actually reason or understand context, they just sort of statistically regurgitate human-sounding text in a way that can fool you into thinking there is real intelligence behind it when there isn't necessarily.
The models do seem to have some real intelligence and understanding, but is it enough for them to be consistently useful tools? That's still an open question. If you ask an AI who Tom Cruise's mother is, it might name someone like Michelle Pfeiffer instead of actually knowing and reasoning about the factual answer.
Alaina: That connects to something I heard an ecological economist YouTuber discuss based on a Boston University study - they talked about the "jagged edge" of AI capabilities. Meaning there are things AI can do that far surpass human abilities, but also simple things humans can do that AI fails at completely. The behaviour is inconsistent in a way that makes it hard to logically determine whether an AI will be good at a particular task or not. You just have to try it.
Hamish: Right, these large language models like GPT-3 use high-dimensional vector representations to encode the meaning of words and concepts, much like how we understand gender to be one dimension of a word's meaning. The models seem to learn these dimensions in a meaningful way - like if you take the vector difference between "king" and "man" and apply it to "woman", you get "queen", suggesting it understands the gender dimension.
But then there are other dimensions the models learn that make no sense to humans at all. We don't know what concepts those strange dimensions are encoding. So there is some real understanding happening, but big gaps in our interpretability of what the models actually know.
Staying on top of all the latest AI research is impossible for any one person. I try to watch YouTube videos from researchers, read analysis from people studying these models, and explore different approaches to make cutting-edge AI usable from an applied perspective.
One area getting a lot of attention is improving the attention mechanisms that allow large language models to maintain context over long sequences of input text. Currently, attention has a major limitation around memory usage - maintaining context over just a bit more history requires vastly more memory. So there's a lot of work happening on resolving that bottleneck.
Alaina: Yeah, going back to what you said earlier about not considering yourself a great predictor of the future - it sounds like in your role, you're very much focused on the short-to-medium term plays for how we can productize and apply AI in practical ways to our work, rather than trying to predict the long-term impacts it could have if it really does become an AGI-level general intelligence.
Hamish: That's accurate. Part of the issue with AGI is that according to documents from organisations like OpenAI, their stated goal is to develop an artificial general intelligence that can do every job a human can do at least as well, or ideally better than humans. And if we make 50% of human jobs obsolete by doing that, the entire concept of money and market economies may no longer make any sense in that world.
There are speculative ideas like "The Singularity" that hypothesise if we do develop AGI, the rate of change will become so rapid that we can't even fathom how different the world will be post-Singularity. At a point, trying to predict that world becomes impossible and not very practical. We could end up in a utopian post-scarcity society where humans are free to pursue whatever they want, like the worlds described in Iain M. Banks' Culture novels. Or it could be a dystopian nightmare controlled by a corporate monopoly on general AI.
So while the idea of strong AI and space exploration holds some romantic appeal for me, I'm much more focused on the pragmatic short-term realities of how we can take current AI/ML capabilities and make them usable for more than just researchers and big tech companies.
Alaina: Right, like taking the complicated state-of-the-art AI models that are often released in a research-oriented way that is messy and hard to use, and creating a clean, accessible interface so the capabilities can be brought to a mass market in a user-friendly way.
Hamish: Exactly, it's as if buying a car required going into a garage where some people had partially assembled engines, others had sketched wheel designs on a wall, but nothing was fully built or integrated. Most people don't want to piecemeal assemble their own car from scattered components - they just want something that works out of the box for their needs.
That's the role I see RUSH AI playing - not trying to design the fundamental AI engines ourselves, but talking to all the companies and researchers pioneering the different engines, understanding the various capabilities, then assembling and integrating those into usable products for our customers' needs.
We're still in that bespoke model of building custom "AI cars" for each set of requirements, because user needs vary so much. We haven't reached the point of having standardised, integrated "models" that work well for most general use cases out of the box.
Alaina: Ha, I like that analogy much better than the typical "ships and shipyards" one for describing software! So then RUSH AI is like the mechanics retrofitting and integrating AI capabilities into custom builds based on each customer's specific needs.
Hamish: Yes, exactly! Though I do think AI will end up being as transformative and industry-shifting as something like the entire Industrial Revolution was, once we get over the usability hurdles.
Alaina: How do you envision AI benefiting our customers, or is that too broad of a question to speculate on?
Hamish: I think in the near-term, the hope is that AI-powered tools and workflows provide efficiency gains, reducing costs for the same quality of output. Or potentially they could provide quality improvements while keeping costs the same.
Ultimately, the goal should be removing tedious grunt work wherever possible so humans can focus on higher-level creative and strategic tasks. Though AI will likely start by augmenting and assisting humans in those processes rather than fully automating everything right away.
Alaina: How will you ensure our AI initiatives remain aligned with RUSH's overall business goals?
Hamish: Since we don't have unlimited capital to invest speculatively, RUSH's AI strategy is very much grounded in how these capabilities can create impact for our existing business and objectives. How can we create technology that empowers humans using AI as a tool? What does that look like as AI reaches more powerful levels?
Alaina: What are some of the risks or ethical challenges associated with implementing AI into products?
Hamish: The big unknown is around data rights, copyright, and ownership issues. Different AI companies have varying levels of transparency around where their training data came from and what licences were attached to that data.
Hamish: Yeah, there is the legal argument from companies developing AI models that the process is sufficiently transformative, so they don't actually need licences for all the individual works in the training data. The outputs are not directly derived from any single work, but from a generalised learning process similar to how humans learn.
Microsoft and others seem to think this legal stance will hold. But a government could also pass laws declaring that AI outputs are derivative works, requiring compensation to all creators whose work went into the training data. Mathematically, that would be impossible - since technically every piece of data used in training is included to some tiny degree in every output.
Sometimes you do get mistakes where one or two source images are overly dominant in an AI-generated output. That's a failing of the training process not learning a properly generalised representation. The intent is for AI outputs to be genuinely new and transformative, not direct derivatives.
Alaina: Right, it's like how a musician's creative works inevitably build upon all the music, teachers, and influences that shaped them, though usually not in a directly derivative way. And just like music, I think the legal case for AI companies is relatively weak regarding claims of violating data copyrights with transformative models.
However, there is a separate moral question around whether we as a society should allow AI to produce unlimited derivative works at a scale that could completely flood the market and drown out the original creators, even if the works are transformative.
We're already seeing cases like authors Amy Webb and Renée Brown who found people passing off books as theirs that had clearly just been trained on their writing by AI models.
Hamish: I agree, that points to issues around ownership and monetisation under modern capitalism, more than being inherent flaws of AI itself. It's people exploiting the technology for nefarious ends, not the AI itself being nefarious. Like, murdering someone with an AI system is still just murder - the AI isn't the root problem there.
Alaina: As someone who has had a career spanning this exponential technology growth curve, let me ask a two-part question: AI is inevitably going to be increasingly applicable for those learning software development, product management, etc.
But we also know resilience, hard work, and persistence breed valuable problem-solvers. Would you hire an engineer who is in high school now and has learned a lot via AI assistance, without necessarily putting in the typically expected "hard yards"?
And the second part - do you think there should be an age limit for using AI assistance?
Hamish: That's an insightful question. The kids growing up now will have to grapple with the realities AI creates regardless. The core issues I worry about for future generations are climate change, misinformation, political polarisation - the toxic stresses we're putting on society and the environment. AI can distort those problems, but doesn't necessarily change the root causes dramatically.
So no, I don't think there should be an age limit on using AI. It's just another tool. As for having to put in the stereotypical "hard yards" - I'm less of a believer that struggle is universally required for developing talent. A lot of my own opportunities came through luck and privilege, like being born in a wealthy country where my dad could afford a computer when I was young, giving me a head start most kids didn't get.
Today's developers are already working at much higher levels of abstraction compared to when I was first learning to code directly to hardware. Back then, everything was low-level - one of the first things game developers had to build was custom loaders to bypass the slow system file loading routines.
Nowadays, developers don't need to know the minutiae of how protocols or operating systems work under the hood. They're building on layers of abstraction handled for them, and just need high-level understanding of APIs and data flows.
So AI assistance is really just another layer of abstraction and productivity enhancement. At the moment, no - relying entirely on an AI coding companion would likely fail to meet quality and requirement standards for professional software roles. But eventually, I'm sure that will change if the AI tools become skilled enough.
The core skills of problem-solving, design thinking, and understanding principles of computer science will still be critical. But the mechanisms of how solutions get implemented could migrate to higher-level AI-assisted processes over time, just as they've continually abstracted upwards through the decades. It's an evolution, not an inherently good or bad thing.
